- Design of Experiments is a framework that allows us to investigate the impact of multiple different factors on an experimental process
- It identifies and explores the interactions between factors and allows researchers to optimize the performance and robustness of processes or assays
- The old conventional approach to scientific experimentation (one-factor-at-a-time, or “OFAT”) are limited in both the number of variables which you can investigate and, critically, preclude investigating how variables interact
- This blog introduces the principles of Design of Experiments, beginning with its origins
- If you’d like to keep learning about DOE after you're done with this article, make sure to check out our other DOE blogs, download our DOE for biologists ebook, or watch our DOE Masterclass webinar series.
DOE Masterclass (Part 1)
What DOE is and how it transforms your biological research
A richer understanding of biological complexity.
What makes a good cup of tea?
A discussion about whether adding milk before or after the tea influences the taste may seem a long way from ensuring that Escherichia coli expresses a particular plasmid, optimizing vaccine formulation and delivery,1,2 or dissecting the intricacies of metabolomics.3
But it's closer than you think.
After all, scientific revolutions can arise from everyday observations: a falling apple inspired Isaac Newton to formulate gravitational theory.
Of all the places for a revolution to start, a tea party in 1920s Cambridge laid the foundations of a statistical technique called Design of Experiments (DOE), which allows researchers to investigate the impact of simultaneously changing multiple factors.
Design of Experiments (DOE): a surprising origin story.
One afternoon some dons, their wives, and guests were having afternoon tea. One lady said she could taste whether tea or milk was poured into the cup first. (Some people believe that hot tea scorches milk, for example.)
The statistician Ronald Fisher, who attended the tea party, devised an experiment to test her claim. The lady was randomly given four cups in which tea was poured before the milk and four where the milk was poured first.
To analyze the interactions between the factors (milk and tea), Ronald devised Fisher’s Exact Test. This determines if any association between the two categorical variables is statistically significant.4
As Figure 1 shows, even four cups of tea can give rise to numerous possible permutations. But this only scratches the surface of tea–making’s complexity.
A perfect cup of tea depends on multiple other factors, such as the blend, brewing time, and the addition of sugar. In other words, making a perfect cup of tea is complex and multidimensional. DOE allows researchers to investigate the effect of changing multiple factors simultaneously.
0= Incorrect; X=correct
Figure 1: Distribution factors assuming that the lady could not distinguish that milk was added before tea (null hypothesis)
In a series of blogs, we’re going to explore the basis of DOE, who should consider DOE, and some ways in which this methodology helps experimental biologists deal with life’s inherent complexity. We’ll begin, however, by going back to school.
School's out... and so is OFAT (one-factor-at-a-time) experimentation.
Our school teachers advocated a one-factor-at-a-time (OFAT) approach to scientific experimentation. So, pick a variable (factor) and vary the value (levels), while keeping everything else constant.
That may be fine in the school lab. Unfortunately, biology doesn’t work that way.
Biological variation, for example, can mean results vary randomly around a set point even in a constant environment. Sample collection, transport, preservation, and measurement systems can introduce further sources of variation.5
DOE helps us understand emergent phenomena.
Biological phenomena, even life itself, are typically emergent. In other words, new patterns and structures appear through the interactions between autonomous elements.6
Every living thing consists of numerous autonomous parts that interact dynamically and unpredictably as part of one or more systems. This means, for example, that you can’t predict cellular diversity by examining nucleotides’ chemical and physical properties.
You also can’t predict the products of cognition by analyzing neuroarchitecture. Emergence is one reason biologists often lack well-developed, robust theoretical frameworks to guide their experiments.
DOE is better for exploring biological complexity.
Most biological processes are complicated, complex, and multidimensional.7 So, changing one factor probably changes something else.
For example, it isn't possible to fully understand the functional consequences of changing a protein's structure without understanding all the contexts in which it appears. Its interactions within biological networks are what really define its function, so even minor changes can produce a plethora of unpredictable down- and upstream effects.
DOE allows the explorations of complex, multidimensional experimental design spaces despite such methodological, biological, or chemical variations.7
OFAT ignores biology’s inherent complexity. It is limited in both the number of variables that you can investigate and, critically, it precludes any investigation of how variables interact.
It’s a bit like trying to analyze the perfect cup of tea by ignoring the temperature of the water, brew time, and blend, and instead just focusing on whether you add the milk first or second.
Figure 2: OFAT may convince you you’ve found an optimum… but it may not be the real one.
Unsurprisingly, OFAT can often identify the wrong system state as the optimum.
Moreover, the lack of well-developed, robust theoretical frameworks can result in unconscious cognitive bias: it’s all too easy to develop OFAT experiments that confirm, rather than test, hypotheses.7
DOE helps avoid unconscious cognitive bias and allows researchers to look behind the curtain of biological complexity to see what’s really going on.
What is Design of Experiments (DOE)?
Design of Experiments is a framework that allows us to investigate the impact of multiple different factors—changed simultaneously—on an experimental process.
DOE also identifies and explores the interactions between those factors. This allows us to optimize the performance and robustness of our processes or assays.
Let’s apply DOE to another simple example: the strawberries you may have with the tea you’ve just added your milk to...
DOE looks at different ranges within factors.
Numerous quantitative factors (e.g. hours of sunlight, grams of plant food, and liters of water) or qualitative factors (e.g. the cultivar) can influence the strawberry crop (Figure 2).
You need to begin by setting a realistic range for each factor. So, testing 1kg of plant food could prove toxic and expensive. Strawberries also need plenty of water to ensure juiciness; applying 1ml of water would be difficult to accurately achieve and, possibly, trigger drought stress responses.
Figure 3: Design of Experiments (DOE) through the example of strawberries. How different factors and levels may impact the yield, weight, and taste of a crop of strawberries
DOE tests many factors at the same time.
The responses we are looking for in this experiment are the yield, the weight, and the taste of the strawberries. You may decide you want a high yield of the tastiest strawberries.
Design of experiments allows you to test numerous factors to determine which make the largest contributions to yield and taste.
Based on this, you can fine-tune the experiment and use DOE to determine which combination of factors at specific levels gives the optimal balance of yield and taste.
You can also compare different levels for given factors, such as whether a cultivar from nursery A produces a higher yield, better taste, or both than a plant from nursery B.
DOE lets you investigate specific outcomes.
Design of Experiments also allows you to investigate specific outcomes (what combinations produce the best balance of yield and taste in a robust way) and reduce variability (define new conditions so the strawberry yield remains the same).
Cost may be another consideration. DOE lets you balance trade-offs, such as what conditions produce the most cost-effective way to achieve the highest yield of strawberries.
DOE Masterclass: Design of Experiments 101 for biologists.
DOE helps reduce the time, materials, and experiments needed to yield a given amount of information compared with OFAT.
As well as these savings, DOE achieves higher precision and reduced variability when estimating the effects of each factor or interaction than using OFAT. It also systematically estimates the interaction between factors, which is not possible with OFAT experiments.
This article offers only a very brief introduction to DOE.
Dive deeper into Design of Experiments:
- Why should I use Design of Experiments in Life Sciences
- When and how to use Design of Experiments (DOE)
- DOE in the real world: when and how to use Design of Experiments
- Types of DOE design: a users' guide
- The DOE process: an overview
- Overcoming barriers to Design of Experiments (DOE)
- 3 reasons why DOE rollouts fail and what to do about it
- Four ways to cut R&D costs with DOE
Well, I’m off for a cup of tea.
Interested in learning more about DOE? Subscribe to our free DOE training course, where you can learn DOE in 6 minutes a day, or catch the full series DOE Masterclass webinar series on our YouTube page.
References
- Ahl PL, Mensch C, Hu B et al. Accelerating vaccine formulation development using design of experiment stability studies. Journal of Pharmaceutical Sciences 2016;105:3046-3056
- Hashiba A, Toyooka M, Sato Y et al. The use of design of experiments with multiple responses to determine optimal formulations for in vivo hepatic mRNA delivery. Journal of Controlled Release 2020;327:467-476
- Surowiec I, Johansson E, Torell F et al. Multivariate strategy for the sample selection and integration of multi-batch data in metabolomics. Metabolomics 2017;13:114
- Bi J and Kuesten C. Revisiting Fisher’s ‘Lady Tasting Tea’ from a perspective of sensory discrimination testing. Food Quality and Preference 2015;43:47-52
- Badrick T. Biological variation: Understanding why it is so important? Practical Laboratory Medicine 2021;23:e00199
- Ikegami T, Mototake Y-i, Kobori S et al. Life as an emergent phenomenon: studies from a large-scale boid simulation and web data. Philosophical Transactions of the Royal Society A: Mathematical, Physical and Engineering Sciences 2017;375:20160351
- Lendrem DW, Lendrem BC, Woods D et al. Lost in space: design of experiments and scientific exploration in a Hogarth Universe. Drug Discovery Today 2015;20:1365-1371
Michael "Sid" Sadowski, PhD
Michael Sadowski, aka Sid, is the Director of Scientific Software at Synthace, where he leads the company’s DOE product development. In his 10 years at the company he has consulted on dozens of DOE campaigns, many of which included aspects of QbD.
Other posts you might be interested in
View All PostsWhen and how to use Design of Experiments (DOE)
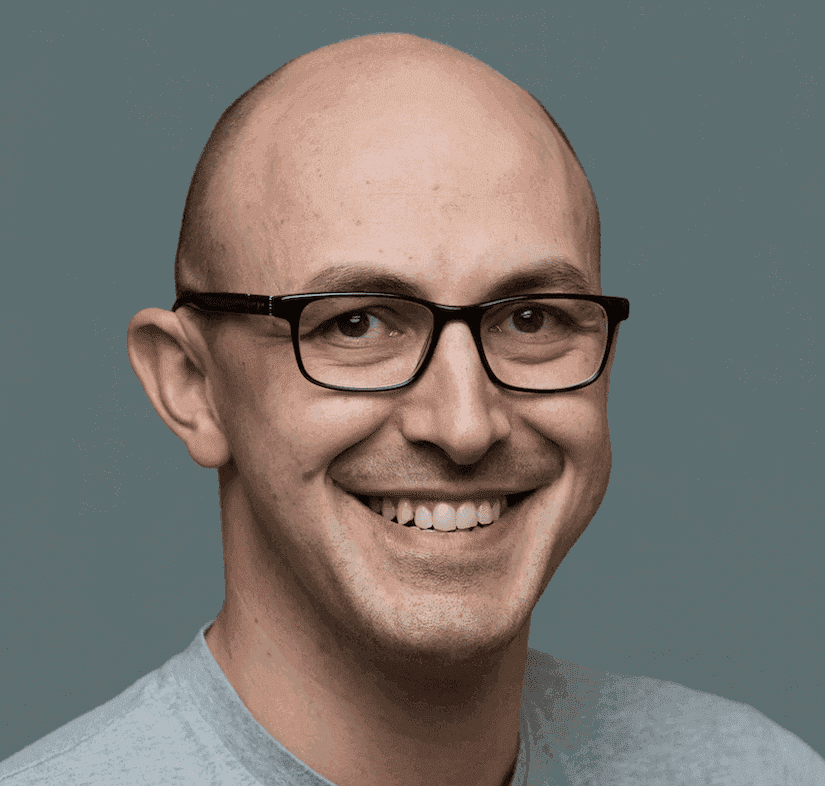
Why Design of Experiments (DOE) is important for biologists
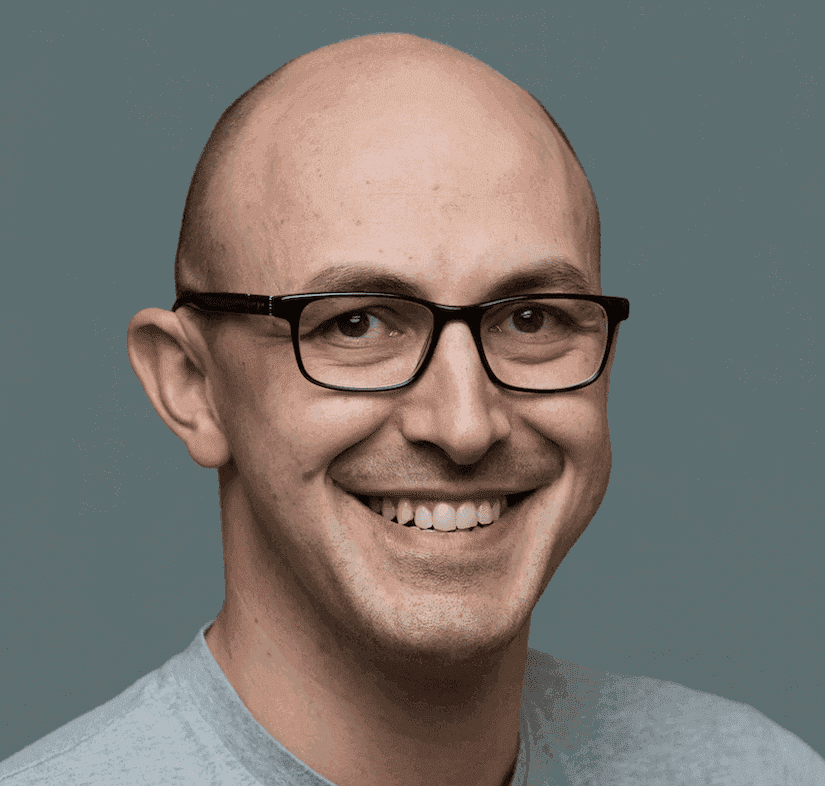
Why Quality by Design (QbD) is vital for pharmaceutical R&D
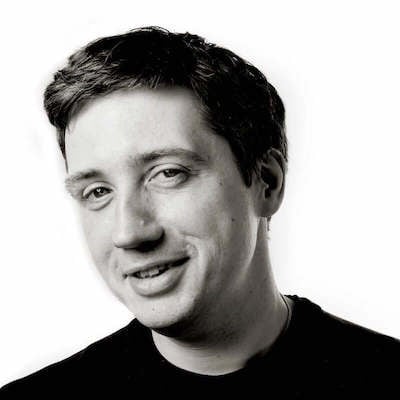