Science advances slowly: It’s more of a drawn-out campaign than a single, targeted strike. And approaching the Design of Experiments (DOE) process is no different.
A DOE campaign needs strategic and tactical planning – but thankfully, much less than the traditional methodology of changing one factor at a time.
Planning a DOE campaign: What you need to know
The DOE process has different stages
Broadly, the process for any DOE campaign encompasses screening, iteration and refinement, optimization, and assessing robustness.
But it’s not a rigid flow. DOE is a flexible process: You can skip steps, and move backwards and forwards as needed.
It’s also iterative—you can end up moving rapidly from your initial ‘thought experiment’ to optimized data.
Before you start, you'll need a goal
Before you begin, it’s important to define what you are hoping to achieve.
Do you want to learn more about your system by characterization? Or are you trying to find the best set of conditions to achieve an optimized output?
The goal shapes your DOE campaign
DOE adapts the experiment to your needs. There aren't any set rules, so the form that your campaign takes—the number and types of stages—will entirely depend on your goal.
So if you’re characterizing your biological space to learn more about your system, then you might achieve this with a single stage—and a very fine grained DOE design, like a space-fill.
But if you’re aiming to find the most optimal conditions in your system, you’re likely to run 3 or more stages iteratively to hone in on those optimal conditions.
Pragmatism trumps perfectionism
At heart, we scientists are often perfectionists. But that mentality can work against us during the DOE process.
It’s worth considering whether you’re simply trying to be "good enough". In other words, are you trying to exceed a threshold?
Let’s say, for example, that you’re growing strawberries. You might be trying to optimize the output—in other words, you want to grow as many strawberries as you can. But you might also be trying to minimize experimental noise. In which case, you need stability in spite of changing conditions.
The question here would be, are you willing to sacrifice some yield for a strawberry crop that remains relatively stable and predictable, despite the weather's vagaries?
The 4 stages of the DOE process, and the steps within them
There are 4 different stage types in a DOE campaign (Figure 1):
Figure 1: The DOE process broken down into stage types: screening, iteration/refinement, optimization, and robustness
As I’ve already mentioned, it’s important to remember that it’s not a rigid flow. You can drop steps as needed, and move backwards and forwards.
Yet with all this flexibility and freedom, you’ll find an unchanging structure embedded at the heart. Within any of these 4 stages, you’ll always take the following steps (Figure 2):
Figure 2: The steps within any of the 4 stage types in the DOE process
Let’s explore each stage of the DOE process in more detail:
1. Screening
What it involves
Screening is the stage in the DOE process where you differentiate factors that are important to your system from those that are less influential. This involves using the maximums and minimums of the ranges for each expected factor, and exploring combinations to identify the importance of single factors and interactions with other variables.
How it helps
Screening is suitable when you have some knowledge of your system, but many factors and you’re trying to identify the select few that have an effect.
Limited prior knowledge can make setting a factor’s range difficult—as it can be all too easy to set the high and low set points for a factor, and entirely miss the range in which it has an observable effect.
But a DOE campaign allows you to revise your choices at each step, which is a big advantage. Even an educated guess is usually a "good enough" starting point.
At this stage, you’re not looking to fit high-quality predictive models. If you only have a few well-characterized factors, then you may be able to bypass screening and go straight to optimization. But fair warning: This can introduce bias. You don’t know what you don’t know. If you can spare the time and resources, it’s always best to start by screening—you might just learn something new that you didn’t know before.
2. Iteration and refinement
What it involves
The iteration and refinement stage involves continuing to investigate which factors are important, and within which ranges.
For example, let’s say we’re still growing our strawberries. Generally, they need slightly acidic soil for optimal growth. Perhaps the soil was initially too alkaline, so you can refine the pH.
Once you have found an acceptable place, then you can reduce the number of factors and explore the ones that remain in much more depth.
How it helps
Sometimes you get lucky, and you hit your success criteria with the first screen. However, in most cases, you are still learning about your system—and the iteration and refinement stage helps you explore your space a bit more to find factor levels closer to optimal.
3. Optimization
What it involves
Optimization means creating a high-quality predictive model to infer optimal conditions for the system. This requires a specific type of DOE design, which investigates the factors in more detail, defined using information from the previous stages. Optimization produces the sets of conditions that give you the best outcomes.
Returning to our strawberries—the model could predict the impact of variations in soil moisture and pH, shade and the proportion of clay, sand and silt in the loam. Exploring a small range and several repeated runs allows you to hone in on the optimal conditions and investigate the noise intrinsic to the system.
How it helps
The optimization stage in the DOE process can help you generate new hypotheses: Do optimal conditions differ depending on whether the strawberries are destined for jam or centre court at Wimbledon? Your optimization run may tell you. Otherwise, you can always go back to earlier stages to find out.
4. Assessing robustness
What it involves
Assessing robustness means determining the extent to which the system is sensitive to changes in levels of your factors. It usually involves running experiments around your optimal conditions—but within the ranges of factor levels that sit in your desired “robustness window”.
How it helps
A robust system will have smaller variability to changes than a more sensitive system. For example, different strains of strawberry may be more or less susceptible to changes in soil moisture, pH, and composition.
The DOE process is very "forgiving', just dive in
That may have been a lot of new information to take in—but don't let that put you off. The beauty of the DOE process is that you don't need to plan in detail up front.
Think about how often your carefully planned experiments have gone awry because of unexpected results or interactions. DOE actually helps you break up your experiment so you can adapt the plan in response to the changes and challenges.
It also steadily increases the return on your experimental investment as you learn more, rather than trying to do everything all at once. Incremental advances are a better bet in the face of significant uncertainty. My final words of advice? The best way to learn is to jump in and use DOE to run your experiments, get some data, and infer next steps. Seeing the results that it can produce first hand will be enough to convince you—and then you'll never look back.
Interested in learning more about DOE? Make sure to check out our other DOE blogs, download our DOE for biologists ebook, or watch our DOE Masterclass webinar series.
James Arpino, PhD
Dr James Arpino, aka JAJA, is a Product Manager at Synthace, where he leads the product development of experiment design and planning. In his seven years at the company he has become an evangelist and expert in transformational multifactorial methods in biology, including DOE.
Other posts you might be interested in
View All Posts3 main types of Design of Experiments (DOE) designs
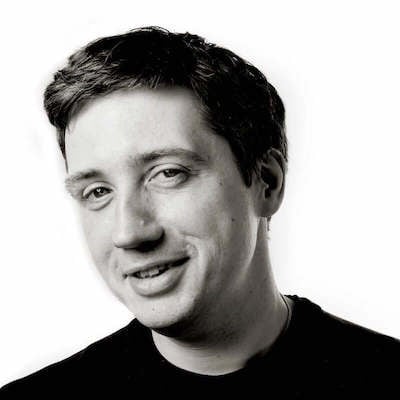
Shaping the Future for Women in STEM: What Can We Do Better?
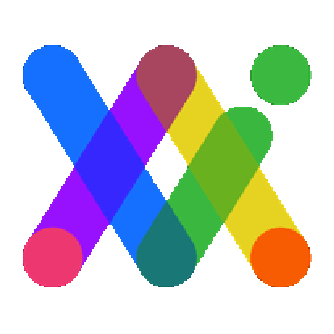
How well does a biologist need to be able to code to automate protocols?
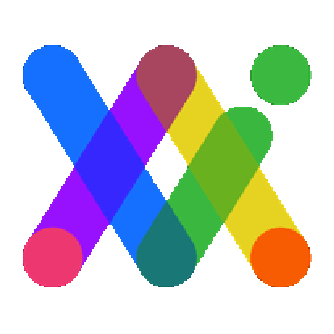