Biology is a notoriously difficult research area, especially for replicating results. To paraphrase from a film that has inspired thousands of people to get into this field: life finds a way (of behaving unexpectedly). Because everything is so interconnected in biology, the one-factor-at-a-time (OFAT) approach is usually taken to investigate biological systems. But what if there were a better way to gain insights into the holistic nature of biology and explore the interconnectedness of various factors while maintaining scientific accuracy?
Well, there is. It’s called Design of Experiments (DOE).
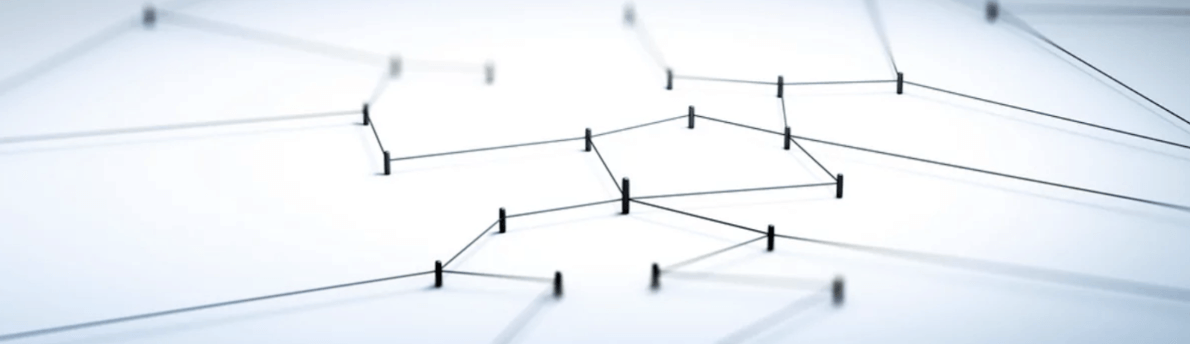
The term ‘Design of Experiments’ can be a confusing one. Of course a scientist is going to design their experiment. In fact, what we are referring to when we say Design of Experiments (or DOE) is a branch of applied statistics that can be applied to experimental design. This systematic method allows scientists to simultaneously investigate the impact of different factors on an experimental process, while also taking the interactions between factors into consideration.
There are plenty of benefits to performing DOE. Compared to other experimental approaches, DOE saves time and resources when performing experiments, whilst providing deeper insight into complex systems.
If DOE is the key to unlocking biological complexity, why is it not used all the time?
Before we dive into that, let’s first look at the more traditional experimental approaches that scientists use to study complex systems.
OFAT vs DOE
In order to fully comprehend the power of DOE, it’s helpful to have an understanding of more commonly used approaches, such as investigating a single factor at a time.
One-factor-at-a-time (OFAT) methods are incredibly common in biological research. One component (factor) is picked at a time and its values (levels) are varied, keeping all other known components constant. In this way, the impact of the selected component can be tested at each variation.
However, experimental optimization using OFAT methods limits the breadth of the possible design space and, by neglecting certain factors or their interactions, often identifies an incorrect optimal state of the system. By testing biological factors in isolation, scientists can be left blind to the interactions between other factors.
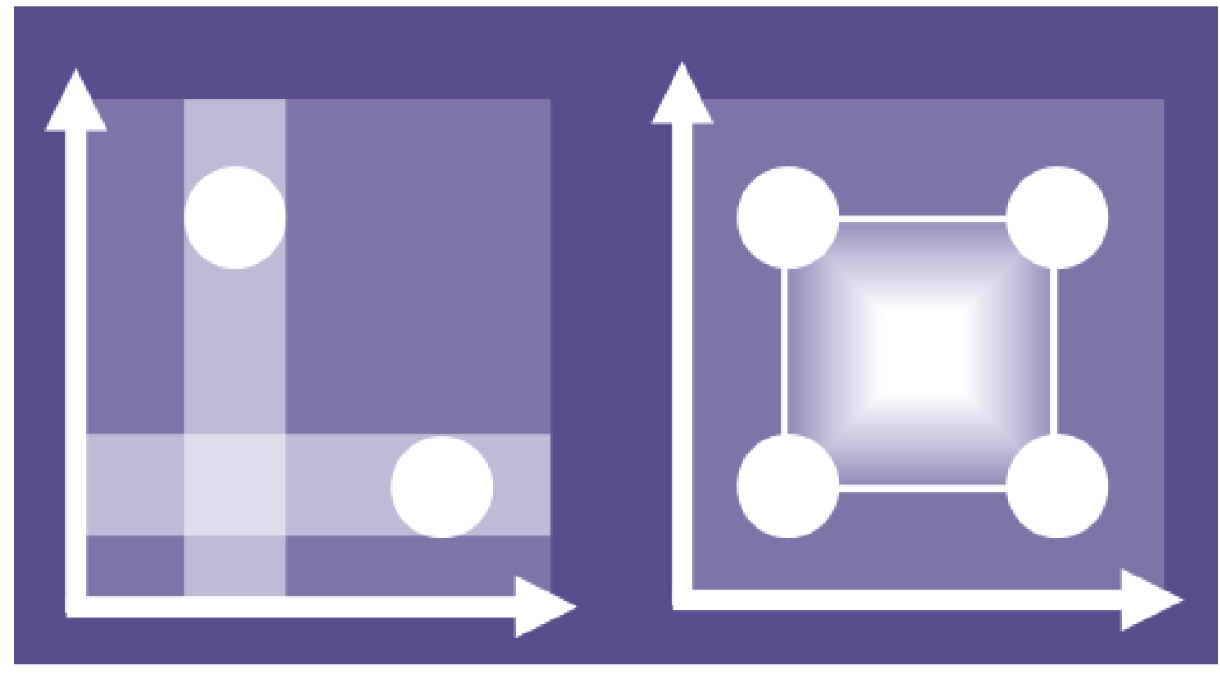
Changing one factor at a time (OFAT, left) means effects are easy to distinguish but there is less information on how factors interact, a critical feature of complex systems. Using statistical techniques to design experiments that explore combinations of factor settings allows their effects to be understood in combination (DOE, right). Optimal results which would otherwise be missed can then be discovered.
In contrast to OFAT experimentation, the systematic structure of experimental conditions in DOE allows researchers to vary and test multiple factors in one go. By simultaneously investigating the effect of many factors on a process of interest, researchers are provided with a more complete understanding of the biological system they are studying.
DOE requires fewer resources for the amount of information obtained, saving on time and materials. By measuring multiple factors at once, you are reducing the number of biological and technical replicates required for a statistically accurate measurement compared to measuring those factors individually.
Additionally, because some factors have a direct or indirect relationship with others, measuring the effect of these factors simultaneously can give better insights into a biological process. These relationships or “interactions” often underpin complex and non-intuitive trends in the data which, in turn, hold key insight into the underlying biological complexity of a system or process.
Interactions between experimental factors are everywhere in bioprocessing but, with traditional experimentation, they are hard to investigate, and often go ignored or unrecognized. In fermentation, for example, pH readout is affected by the temperature of the medium and will shift as temperature changes, even before the medium is inoculated. By using a DOE approach researchers can pin down crucial interacting factors and gain crucial understanding and insight into how they can be exploited or controlled to improve system performance. When working in highly complicated systems and processes, such as in the production of biological therapeutics, DOE is the best approach to optimizing a process.
Even with all its benefits, many biologists still don’t perform DOE. This is for a number of reasons. DOE can be daunting to execute when the interactions of large numbers of factors need to be measured. Many biologists are still unfamiliar with DOE if they didn’t study it or haven’t used it before, and it may be hard to know where to start.
Getting started with DOE
As with anything, there can be a learning curve to setting up and starting to perform DOE.
DOE can be difficult to plan and analyze; however experimental execution of a DOE can be particularly challenging, especially for those less comfortable with automation. DOE can be performed manually for two or three factors simultaneously, but as the number of factors increases or if you have liquid handling robots to carry out more complex experiments, the planning and attention to detail required to execute complex DOE designs becomes a significant burden, you will need to use specialized software such as JMP to help design and model your experiments, and build a statistically accurate picture of your process. Whatever software package you use, there is plenty of support and information to help you design and analyze your experiment.
One benefit of the COVID pandemic is that companies have put a lot of demos and resources online. You can see DOE in action with liquid handling and automation here, and how software tools can help design and carry out DOE experiments. Another great resource that we highly recommend for people starting out with DOE is the book DOE Simplified: Practical Tools for Effective Experimentation by Mark J. Anderson and Patrick J. Whitcomb.
Let us help you get started with DOE. Join our DOE masterclass webinar for biologists.

DOE and Machine Learning
Machine Learning (ML), whereby computational algorithms interpret complex data, is a methodological approach to solving optimization problems when there is a lot of data available. DOE can help ML approaches become more effective by finding the optimal algorithmic parameter settings, while ML can support DOE by better detecting the effects of factors and their interactions. Biological experimentation can be expensive, but through the use of DOE, coupled with ML, it may be possible to build Machine Learning capabilities using smaller (and less costly) data sets. This is especially useful as experiments scale up and the amount of data generated is difficult to collect and process manually.
Liquid handling technologies allow us to consider more complex DOE experiments than ever before as they transcend the human limitations of carrying out physical work. This results in much more data captured by the software, as well as metadata that contextualizes the main data points of the factors under examination. By leveraging the power of ML in data analysis, the effect of the metadata can be considered in addition to the main data points in how outputs are affected by a process.
Transforming the biological research landscape with DOE
DOE is a powerful statistical and experimental design tool that allows biological researchers to make their processes more defined and predictable. The methodology is well suited to automating liquid handling and an array of software tools exist to help translate DOE designs into viable experiments. The data bottleneck can be addressed manually for small-scale DOE designs, but new software tools like ML can tease out new insights from the data allowing for more predictable and accurate research in the traditionally unpredictable field of biology.
DOE is already a cornerstone of industry standards supporting Quality by Design principles and the adoption of Computer-Aided Biology tools in this space is well underway. DOE and supporting CAB technologies are poised to transform the biological research landscape, uncovering new insights from data and ensuring biological research is more robust and precise than ever.
Learn more about DOE from our in-house experts by clicking here.
Other posts you might be interested in
View All PostsWhy Design of Experiments (DOE) is important for biologists
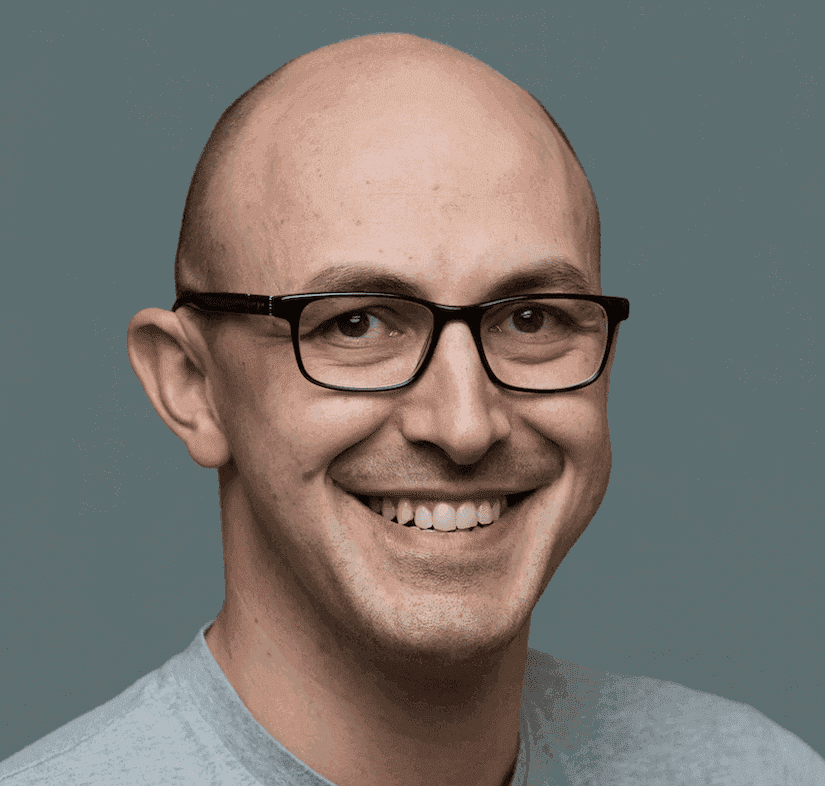
Leveraging the Power of Design of Experiments (DoE) For Optimizing Growth Media Composition

Three Women Pioneering a New Approach to Biological R&D
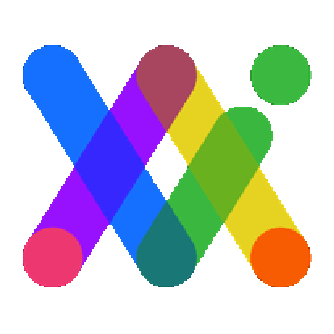