Experimental data generation is key for FAIR data strategy
As life-science and biopharmaceutical companies evolve to become digital-first companies, how a company derives and utilizes its data has become an important differentiator. As such, it is essential that not only organizations but also the software and hardware vendors providing digital solutions in this field invest in infrastructure to preserve the utility of data being handled by their systems, from raw data generation to curation and long-term storage and management. The utility of a data set is proportional to its quality and application, and whilst data can be enhanced retrospectively, it is far easier to invest in producing quality contextualized and structured experimental data at the point of creation.
At Synthace, our platform is being built to automate the generation of a rich set of data and metadata and in the process provides a high level of traceability and context for every experimental workflow. This allows the Synthace platform to standardize and automate the data journey across these workflows and produce data sets that can be pipelined into the wider informatics and analytics ecosystem.
As part of our roadmap, we are continuously monitoring best practices, such as the FAIR principles, and identifying areas where we can strengthen our approach to improving the data quality of our customers. Here are some of the ways that we currently align with the FAIR principles:
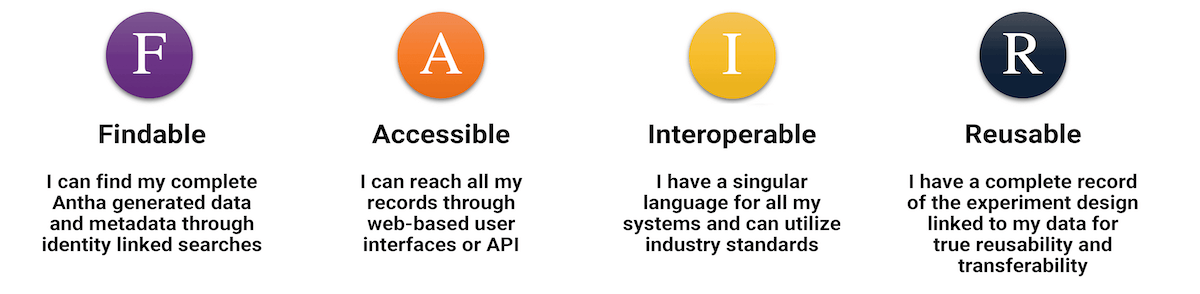
Findable: All of the rich data and metadata generated by Synthace is stored as a coherent data record containing both data and the associated metadata together. This data and metadata can be searched by identifier when the correct access is granted.
Accessible: Customers are able to access experimental data and metadata by its simulation/execution ID through the Synthace interface which is provided over a standardized communications protocol.
Interoperable: Synthace data and metadata follows an implicit internal ontology to ensure consistency within the platform. Synthace is committed to provide data and metadata in industry-standard formats required to make that data shareable and accessible and to adopt industry standard ontologies as they emerge.
Reusable: For each run, information is stored on when and how the run was performed in order to maintain detailed provenance. All run data and metadata for an experiment in the Synthace platform are stored with a rich collection of attributes.
We look forward to continuing to evolve and push forward new efforts for quality and standardization with our customers and with fellow members of the Pistoia Alliance and Allotrope.
Other posts you might be interested in
View All PostsMiniaturized Purification with Synthace and Tecan Te-Chrom™: Uniting Robust Execution With Flexible Planning and Comprehensive Data Analysis

A sample-oriented approach to experiment automation
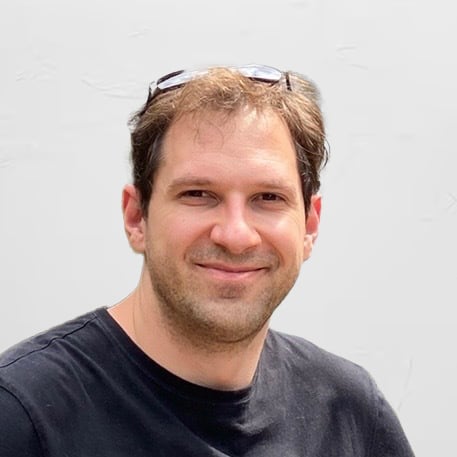
Why biology needs a digital model for experiments
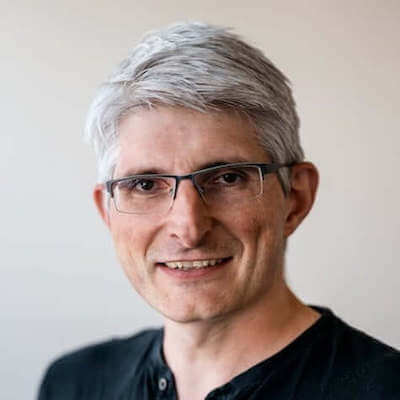