High Dimensional Experimentation: a new era for assay development
When we first started working with AstraZeneca, we thought it would be much like any other project: get design of experiments (DOE) up and running in their assay development function and watch as a team of great scientists gets to work. But, in the end, it went way further: a much deeper scientific transformation than either we—or the team at AstraZeneca—could have expected.
It also led to the advent of a new methodology. But I’ll come back to that.
Historically, DOEs have been hard to run. This means that they’re usually only used by a handful of DOE fanatics, determined to make this powerful methodology work. There’re many reasons why it’s been so hard to run them in the past, from misunderstandings about what it is, to complex automation programming, all the way through to good old-fashioned resistance to change.
The difference with our platform, though, is that it makes DOE that much easier to get up and running in a lab. Import your design (from JMP, for example) and you’re automating within minutes, then pulling all your data automatically afterward. Even so, with the reputation DOE has for being “difficult,” we’re happy if we can just get a team comfortable with using DOE at all.
But AstraZeneca? What they were able to do has blown us away.
Running impossible experiments
At SLAS Europe earlier this year, I shared a stage with Dr. Helena Peilot Sjögren, Principal Scientist in Discovery Biology at AstraZeneca, who led the project at AZ to introduce and roll Synthace out. As she made her way through her presentation, she paused to clarify an important overall difference: “This platform enables you to run experiments that were previously impossible.”
One team found a way to run an assay with 50% less reagent for the same quality assay. Another explored the entire design space of one assay—a buffer screen with 9 factors, generating full binding curves in 75 unique conditions—to definitively confirm that buffer choice had no effect. A negative result, but a clear one that allowed that project to move on rapidly. Another example had a substantial improvement of Z’ (the highly stringent signal-to-noise measure used for high-throughput assays), and a doubling of the assay window.
There are dozens more examples, none of which I am at liberty to share here. But I’ll just say this: in all my time as a biologist, I’ve never seen anything like it. It is crystal clear to me that we are now talking about a new class of experiment altogether.
We call it High Dimensional Experimentation, or HDE for short.
What is high dimensional experimentation (HDE)?
We’ve already spoken at length about the difference between “one factor at a time” and DOE. With the former, we hold all variables constant but one, measuring the response before doing the same with another. It’s simple enough, but it’s an approach that makes the fatal assumption of no interaction between factors. But this is biology we’re talking about—of course there are interactions!
OFAT methodology: cross sections (purple lines) are made through design space, missing any interactions between factors
With DOE, we change multiple factors at the same time to study those interactions and explore design space a lot more effectively. With it, we see the bigger picture and uncover things we otherwise would have missed. So what’s so different about HDE? Is it just a souped-up version of DOE? Yes and no.
A “classic” DOE design favors highly efficient designs, used over several iterations to explore a broad, albeit inexhaustive, design space. With HDE, we blow caution out of the water. When we have access to (and integration with) equipment that can run experiments with 100s or 1000s of runs with volumes as low as 3-20 μl per run, why not explore the entire space in a single shot?
DOE (left) requires multiple iterations to reach a local optimum. HDE by contrast maps the whole space in one experiment
Usually, the complexity of planning and running this kind of experiment would be completely prohibitive, but AstraZeneca were able to use Synthace to generate all the instructions needed to run these experiments on dispensers like the Dragonfly Discovery, the Gyger Certus Flex, and the Beckman Echo.
HDE isn’t all about execution, though. To succeed, it must include integrated experiment design and planning, as well as data capture and structuring. After all, if you make your experiments dramatically more complex and sophisticated you will generate a large volume of more complex data and metadata. Manual structuring of these complex, fragmented datasets is possible but it takes a large amount of tedious, error-prone work.
With Synthace, the entire experiment lives in one platform from design, to execution, right through to data aggregation.
Combining these capabilities with the right hardware and biological use cases allows us to work with volumes from microlitres to nanolitres, and drastically increase the possible space that we can explore.
How High Dimensional Experimentation is changing assay development and drug discovery
Assays are fundamental in all drug development. They are our window into a world of delicate interactions, telling us which molecules might save lives and helping us discard the ones that do nothing or, worse, hurt the patients we’re trying to help.
Without assays, we have no drug discovery. Without good assays, we make slow and frustrating progress. With good assays, we make discoveries. With HDE-accelerated assays, though? The benefits are enormous:
- Dramatically faster assay development- knocking months off the critical path for drug discovery
- Full mapping of design space, yielding higher performing, more robust assays
- Lower cost
- Higher quality
- Faster time-to-insight
Consider it for a second: if you could make some of the improvements to your assays that I listed above, what impact would it have on your preclinical trials? What would it mean to your overall pipeline?
What comes after HDE?
So far, we’ve shown a visualization of what this looks like in a single biological design space. Now imagine this same work across ten more. Now in a hundred more. Now in an unlimited number of ways, with context-rich data and metadata structured and formatted, sent to the cloud, and plugged into artificial intelligence and machine learning tools.
An image of what's to come: multiple HDE datasets, collated and—potentially—fed into AI/ML tools.
We might imagine a future where assay development starts with a single query, written in plain English, that prompts an AI to leverage unfathomable data and computing power to design a near-perfect assay in seconds. Maybe it sounds too good to be true. This is only the beginning, after all. But what a beginning it is.
If you’re interested in learning more about using DOE for Assay Development, you can watch a brief interview Dr. Sjögren gave to SelectScience afterward. You can watch it here.
Markus Gershater, PhD
Markus is a co-founder of Synthace and one of the UK’s leading visionaries for how we, as a society, can do better biology. Originally establishing Synthace as a synthetic biology company, he was struck with the conviction that so much potential progress is held back by tedious, one-dimensional, error-prone, manual...
Other posts you might be interested in
View All PostsImplementing Design of Experiments (DOE): A practical example
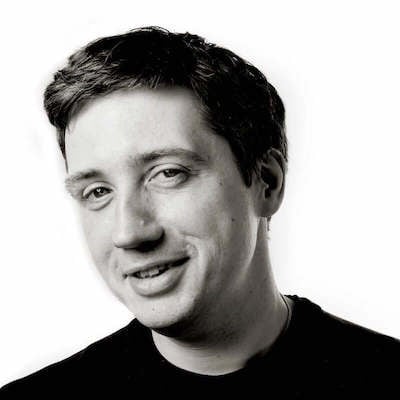
How to Make High Throughput Design of Experiments the Norm in Assay Development
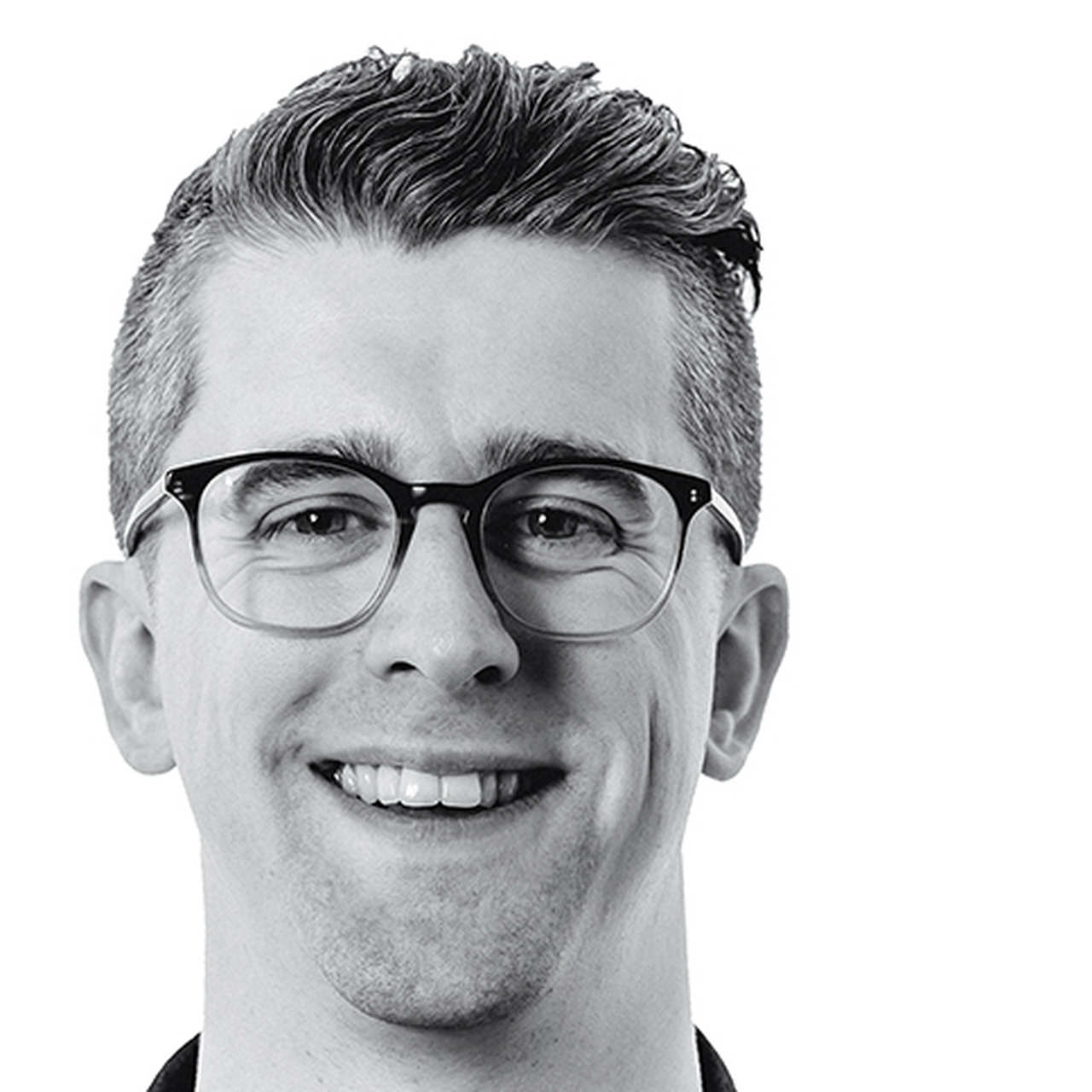
Why biology needs a digital model for experiments
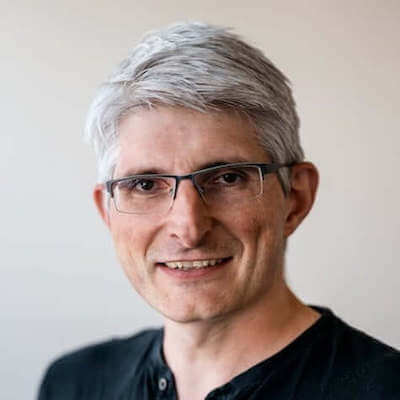