Here’s Why You Should Stop Doing Science Like You Were Taught in High School: Part 2
Read “Here’s Why You Should Stop Doing Science Like You Were Taught in High School: Part 1”
From One-Factor-At-A-Time to Design of Experiments
One-factor-at-a-time (OFAT) methods were widely adopted by scientists to simplify the study of complex systems. One component (factor) is picked at a time and its values (levels) are varied, keeping all other known components constant. In this way, the impact of the selected component can be tested at each variation.
However, experimental optimization using OFAT methods limits the breadth of the possible design space and, by neglecting certain factors or their interactions, often identifies an incorrect optimal state of the system.
Design of Experiments (DOE) is a statistical method conceived with the caveats of OFAT in mind [1]. It allows scientists to investigate multiple different factors at different levels at the same time, while also taking their interactions into consideration. This approach greatly broadens the experimental or assay design space and provides insights that cannot be achieved otherwise.
The Problem with Performing DOEs Manually and The Pressing Need for Flexible Lab Automation
The number of DOE runs increases exponentially with every factor that is varied, making manual execution difficult or virtually impossible beyond 2-factor experiments.
For example, to execute a DOE optimization of buffers used for construct assembly, there are different factors that could affect the final result, i.e. the transfection efficiency of target cells. These factors can include DNA quantity, buffer component concentrations, enzyme concentration, temperature, time of incubation, and others.
We may choose to vary only the DNA quantity across different levels to see its effect on transfection efficiency. Then, the effect of enzyme concentration as well as the interaction between the enzyme and DNA concentrations (an extremely important one) are neglected, and important information is lost. To avoid this, we may consider varying enzyme concentration in parallel with the DNA concentration. However, that still may not be enough – the buffer composition also needs to be taken into account and optimized for the new enzyme quantities used.
Therefore, if we take into account all the interactions between different factors that affect the final outcome of the experiment, the number of different combinations to be considered rises exponentially and DOEs can quickly become overwhelming. But the insight gained is well worth the effort.
To overcome the challenge of this overwhelming complexity, in DOE-driven experimental design, most execution steps are carried out using lab automation, namely liquid handling devices.
However, while allowing scientists to scale up the testing of different factor levels in DOE assays, lab automation also comes with its own limitations. It often relies on device-specific vendor software, where programming complex sets of liquid handling instructions, such as those involved in DOE designs, requires a lot of programming knowledge and poses a high barrier to entry for biologists.
Furthermore, the lack of flexibility in coding lab automation means that even minor changes to DOE designs require users to re-program the entire sets of sophisticated execution steps within the vendor software, which can become a huge time sink for scientists that want to carry out their experiment.
With these problems in mind, we developed our own automation platform, which offers a codeless, user-friendly interface for designing, optimizing, and executing sophisticated experiments, such as DOE optimization assays.
The Synthace platform has been successfully deployed in a number of DOE projects, both within Synthace and across the wider industry:
- Learn about the sophisticated space-filling DOE we did in collaboration with SPT Labtech, where we performed a 3,456-run DOE in under 8 hours
- Read about the automated ‘closed-loop’ DOE we performed in collaboration with LabGenius and their EVA platform to drive protein design evolution, saving ~70% time and providing them the flexibility to autonomously design, plan, and physically execute process optimization as part of their end-to-end platform
- Check out our application note, where we performed a DOE optimization of 7-part construct assembly, going from 35% to >95% transformation efficiency in three iterations
Synthace-Powered Multifactorial Buffer Optimisation for Construct Assembly Assays
Construct assembly assays are used for inserting desired DNA sequences into a vector, usually a bacterial plasmid, to form genetic constructs encoding specific proteins for further bioprocess. The more DNA parts are to be inserted, the more time-consuming and error-prone this process is. Therefore, it is important to optimize the assay prior to large-scale execution to ensure the most suitable conditions and maximize the chances of experimental success.
When optimizing construct assembly reactions, factors that can be varied include concentration or quantities of the buffer, enzymes, DNA components, or conditions like incubation temperature.
In this demo, we demonstrate how the Synthace platform can be used for seamless execution of a DOE design for buffer optimization in construct assembly assays. We were able to vary 5 factors at 3 levels each and at 2 temperatures, which amounted to a total of 486 reactions.
To compose and test such complexity would be impossible using manual approaches or most alternative vendor software.
Read “Here’s Why You Should Stop Doing Science Like You Were Taught in High School: Part 1”
Other posts you might be interested in
View All PostsHere’s Why You Should Stop Doing Science Like You Were Taught in High School: Part 3

Here’s Why You Should Stop Doing Science Like You Were Taught in High School (Part 1)

How to Work in Your Lab… Without Being in Your Lab
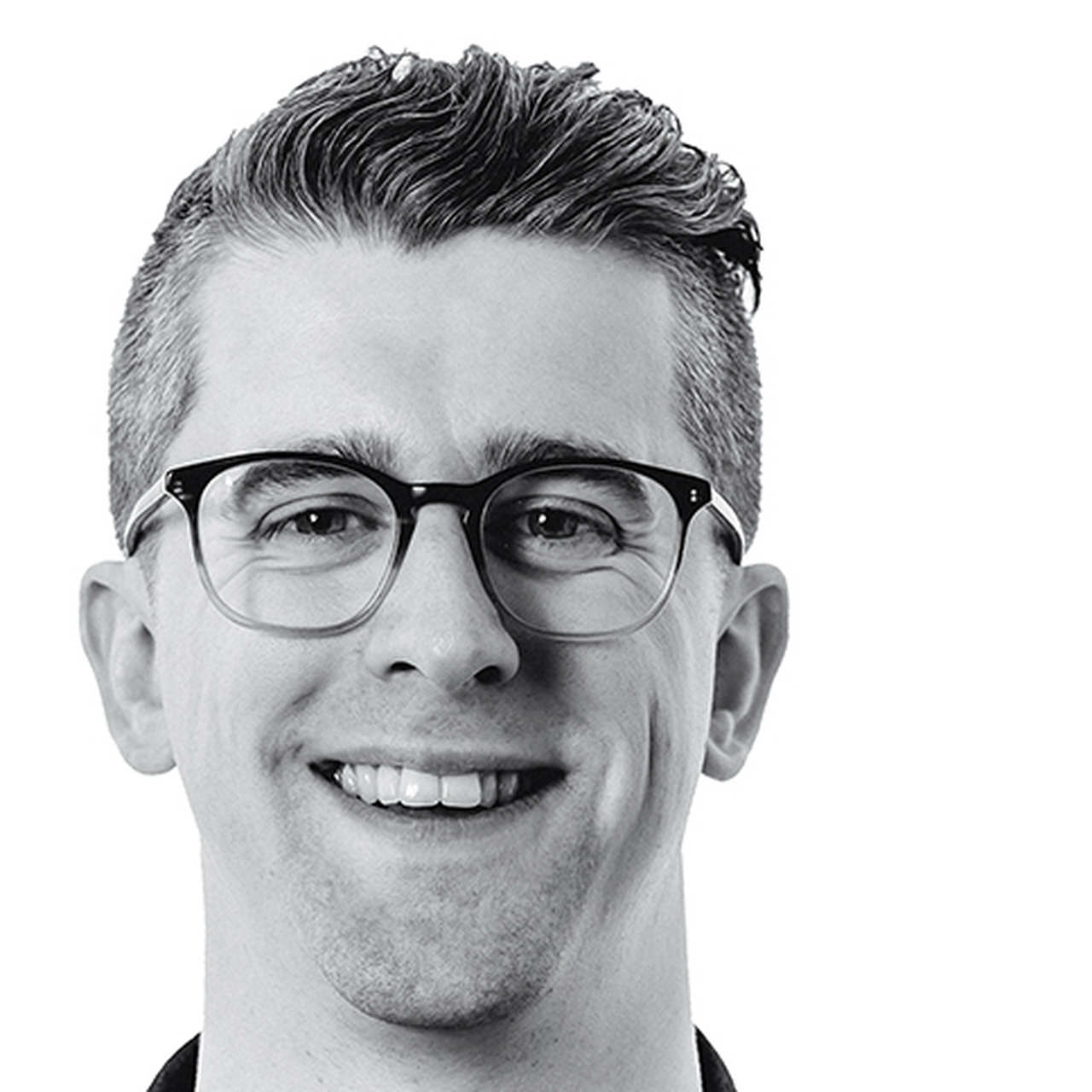