In this interview, we sit down with Dr. Susan Shiff MBA to discuss how data is being obtained, analyzed, and used from across the healthcare system to extract insights that deliver meaningful patient outcomes. What we discover is that the healthcare industry faces a common set of data challenges: whether that’s in the discovery lab or at the point of care.
Susan is an Independent Non-Executive Director at Synthace; and SVP and Head of the Centre for Observational and Real-World Evidence at MSD (known as Merck & Co., Inc., Kenilworth NJ USA). She is also a non-executive director at Synthace.
To start, would you be able to give our readers an overview of your background?
I joined MSD in 2014 as Senior Vice President and head of CORE or the Center for Observational and Real World Evidence. Prior to that, I spent seven years at Pfizer, including several years as a Vice President in the areas of outcomes research, epidemiology, health economics, and access and pricing. I started my career at the U.S. Centers for Disease Control and Prevention (CDC). I received my Ph.D. degree from UCLA and my MBA degree from Cornell University.
You mentioned real-world data? Could you just give an outline of what real-world data and real-world evidence are?
Real-world evidence (RWE) and real-world data (RWD) are indeed different. RWD are data related to a patient and their health care status and the data that is routinely collected during the course of a patient's interactions with the healthcare system. Data sources include a patient's billing records, their electronic medical records, or their pharmacy claims. RWE, on the other hand, are the insights that we've gained from analyzing RWD.
How is this data-driven real-world evidence approach different from the way things were traditionally done? Or is this just a continuation of existing methods and approaches?
It is a different approach as we are looking at the benefits and risks of health technology in the real world, not in the structure of an experiment or clinical trial.
Within the real world setting, there are terabytes of health care data being created every day. Data sources include claims data, electronic medical records, genomics information, medical test results, wearables, and patient-reported outcomes. The availability of these data allows us to answer complex questions about the healthcare system and about the effectiveness of health technologies.
The methods we apply to these data have also advanced and include AI, natural language processing, and deep learning. Using these methods, for example, can help us and other stakeholders predict patient response to a health technology or identify subpopulations that are more likely to respond to treatment.
Do you feel like real-world evidence is a complement to some of the data-driven therapeutic discovery approaches?
Absolutely, both the data and the methods complement drug discovery. For example, RWD can help us identify responder cohorts to further focus our development strategy. The application of machine learning to the complex design of experiments can focus and speed the experimental process.
Do you feel it's the data access or it's the tools that are perhaps where the focus needs to be?
It's both access to the right data and utilization of advanced methods. Both the data and the analytic methods can help us both Interpret and revolutionize biology, medicine, and overall healthcare.
How do you see this area evolving, and maybe what are some of the risks and opportunities you see over the foreseeable future?
There will be broader and deeper real-world data available and access to these data will most likely be broad. As these data become available to both scientists and non-scientists, a risk is that those without the appropriate training in the scientific method will publish results that are spurious or could hurt patients by steering them to the wrong or suboptimal treatments.
Other posts you might be interested in
View All PostsMeet Thomas Pesnot, Drug Discovery Services Director at Concept Life Sciences
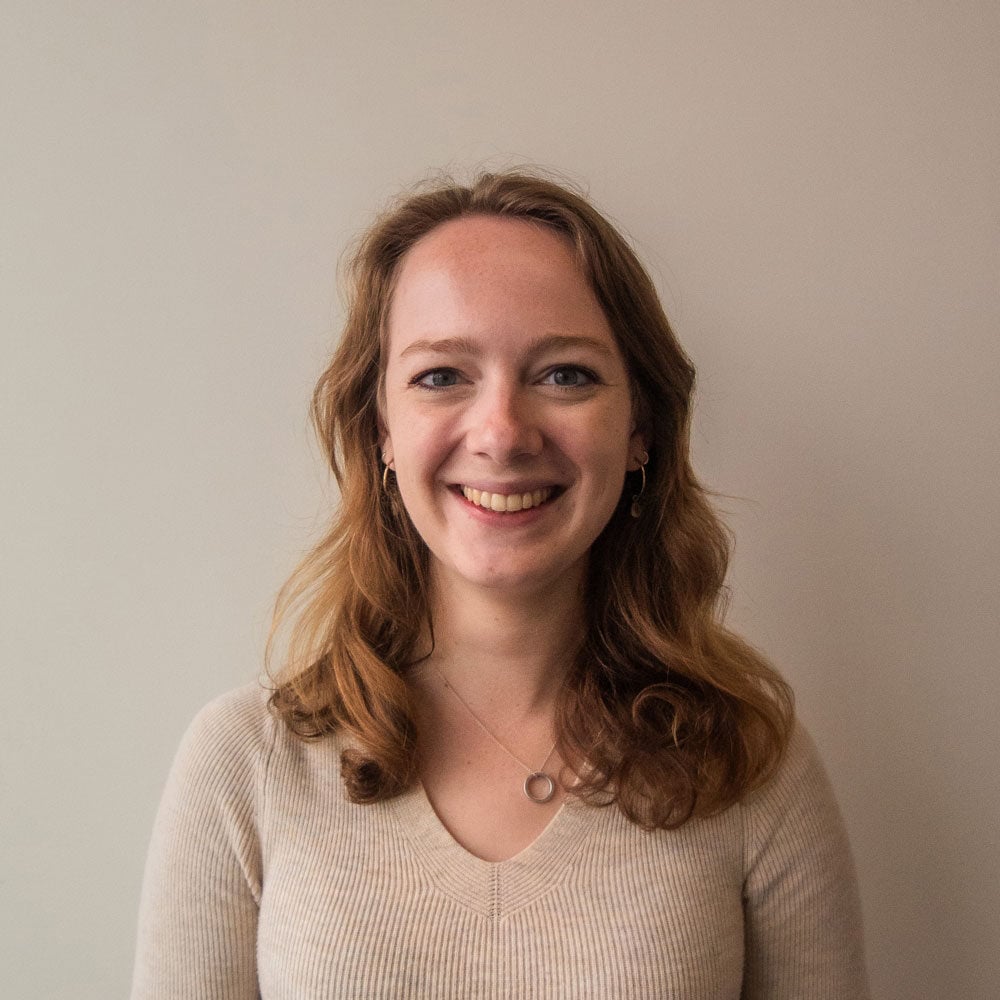
The horror of climate change is here, and labs must do their part
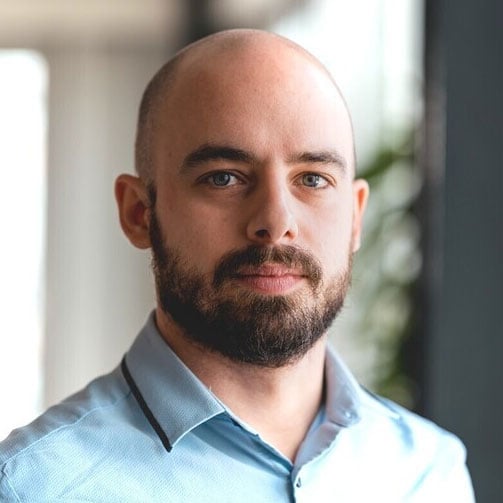
Synthace Expert Interviews: Cell and Gene Therapies with Dr Damian Marshall (part 2)
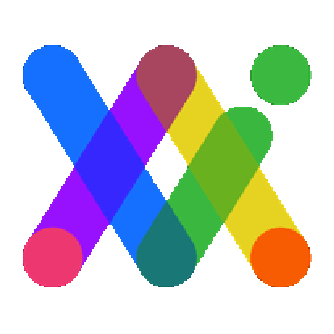